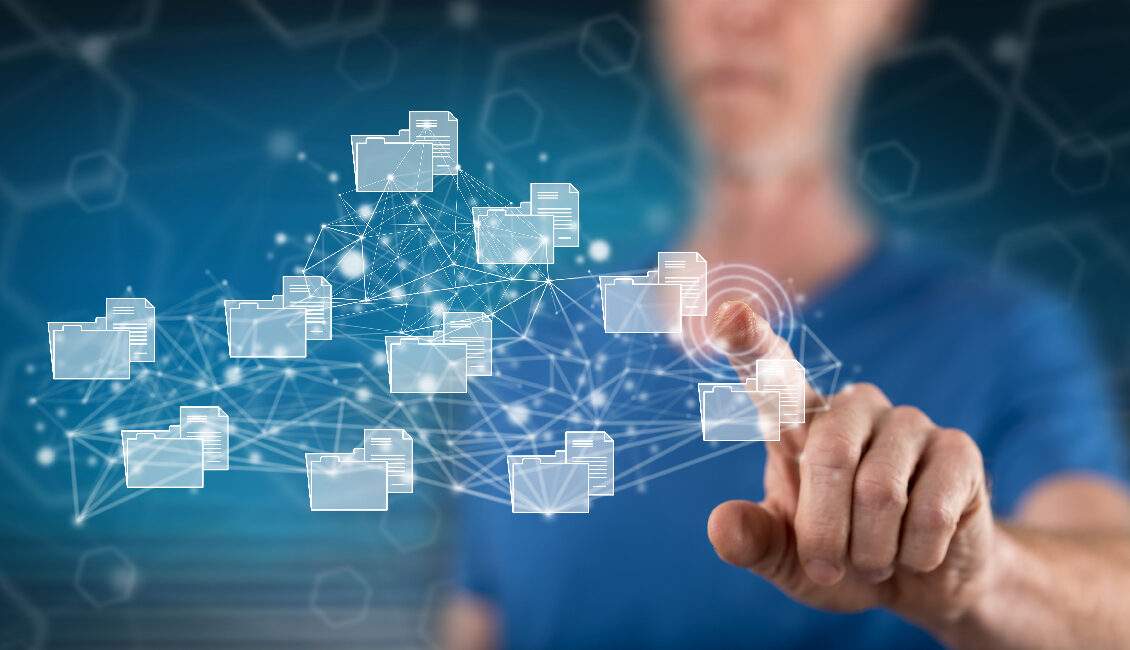
The Seven Deadly Data Sins
19 min read
Abstract: Organizations routinely try and solve all their problems technologically at the expense of the business. There are seven problems, known as the “Seven Deadly Data Sins”, that organizations encounter on their way towards getting the maximum return out of their data. This article presents discrete and objective goals organizations can bring to pursue based on the guidance described in this paper:
- Establish data management knowledge, skills, and abilities;
- Establish qualified hiring panels;
- Identify your organization’s first chief data officer;
- Remove barriers to leveraging data
- Begin to think in a data-centric manner;
- Establish a programmatic way to share data;
- Establish protocols for orchestrating the data program with IT projects;
- Establish a process to sequence the implementation of the data strategy; and
- Recognize and address deeply-rooted organizational culture.
Introduction
Organizations with a willingness to change and the requisite talent still can’t implement a data strategy unless the organization:
- Understands the underpinnings of data-centric thinking.
- Obtains qualified leadership for data initiatives.
- Separates data and software development.
- Sequences IT projects and the data program properly.
- Manages expectations.
- Sequences its data strategy appropriately.
- Addresses cultural and change management aspects of data programs.
The capabilities expressed in these sins constitute the foundation upon which data projects, initiatives, technologies and strategies are built. Because the foundation—the sum of these capabilities—is only as strong as its weakest component, it is imperative for organizations to overcome these barriers early in their work. Failure to eliminate all of them will prevent organizations from successfully implementing a data strategy. Each of the Seven Deadly Data Sins is described in detail below. This work is the beginning of a longer set of evolutionary steps toward managing enterprise data as a strategic asset.
Data Sin No 1: Failing to Understand Data-Centric Thinking
The ideas and motives that form the foundation of data-centric thinking require that certain topics become cornerstones of a required academic data curriculum. Currently, colleges and universities do not consider these concepts as base or core concepts; most are not taught at all, and it will likely take decades to successfully introduce these ideas in traditional academic thinking. When learning institutions ultimately do accept and incorporate these ideas into their curricula, they will begin to graduate knowledgeable data professionals. Until then, however, organizations will have to rely on professional organizations, like DAMA International, the Association for Federal Information Resource Management (AFFIRM), the International Association for Information and Data Quality (IAIDQ), Capability Maturity Model Integration Data Management Maturity (CMMI/DMM℠), International Society of Chief Data Officers (ISCDO) and others to fill this knowledge gap and increase the maturity of data practitioners.
Like other fields of study, data management requires a solid, considerate, and comprehensive educational foundation. Just as you can’t build any structure on a poorly designed foundation, organizations need solid foundational data management practices if they are to effectively and efficiently leverage data. For example, organizations need to have standard ways of acquiring, processing, storing, and sharing data assets. Without these core services and capabilities, organizations at every level will continue to invent their own ways of doing things to the detriment of the enterprise. It is incumbent on every organization and its leadership to ensure that all participants, not just the data management team, increase the organization’s data literacy and understand these principles to successfully leverage data. This is not an easy task because few people within leadership circles are qualified to manage data, consequently leaving the organization in a delicate and precarious situation with its leadership not knowing what they truly need to know. Until academia develops a data curriculum, organizations will bear the full cost of poor data literacy.
Compounding matters, organizational leadership does not realize that data management work is grounded in enterprise architecture and systems engineering, and it can’t be combined with software development or other information technology project-based work. When organizations work this way, two very costly things happen: (1) data management is treated as a part of individual projects and (2) business expects IT to do it correctly. What should be done instead is for data management professionals to work together with businesses and abstract their needs into actionable data requirements for the enterprise.
In the end, the point is really quite simple: organizations can’t implement a data strategy without first becoming more educated and developing the knowledge, skills, and abilities required to transform the organization from being IT-focused to data-focused. Remember, too, there is no shortcut, no quick-win for developing these capabilities. Organizations need to invest time, money and resources to help develop a foundational capability that would ultimately mature into a sustainable enterprise set of services. At the same time, organizations need to carefully level set expectations letting leadership know this is something that will mature over years and sometimes over decades. The most important lesson, though, is that we can’t currently trust the educational system to supply foundational knowledge about this important field, and organizations will not be able to rely on it for many years to come.
Data Sin No 2: Lacking Qualified Data Leadership
For nearly every executive position today, there is at least one educational path for students to follow. For instance, if someone were interested in becoming a chief executive officer, colleges and universities offer numerous degree programs that would prepare the student for the challenges of running a company. The most obvious example is graduate degrees in business administration, more commonly known as a Master of Business Administration (MBA). Likewise, if someone were interested in becoming a chief financial officer, schools again offer numerous degree programs to support this need. However, if someone were interested in becoming an enterprise data executive, colleges and universities offer no specific or holistic program of study. Instead, some schools offer selected topics scattered across many different programs most notably within the library and information science schools.
Because our educational system treats data as a technical discipline, the knowledge base needed to manage large, complex issues and activities associated with data simply does not exist in our academic institutions. So, while organizations are rapidly realizing they need someone who is exclusively focused on data, there is no viable candidate pool available to them. In many instances, organizations basically appoint someone they believe mostly closely represents their needs, and organizations generally default to information technology experts. This means the newly-appointed data leadership views every data issue as a technical challenge, one requiring technical solutions. In the end, lacking qualified data leadership prevents organizations from finding and securing qualified executive leaders who are well-versed in the topic of data. Because the EDE role is still new and lacking an academic and credentialed foundation, organizations are largely unaware of the business process, architecture and engineering requirements needed to successfully reuse and optimize their data assets.
Sadly, today, data management uses few scientific, research, architectural, or engineering principles. It is largely a collection of relatively new and immature industry standards and courses sprinkled across various academic programs. Thus, finding organized data management knowledge is difficult to do, and little of this knowledge exists in most organizations. With the exception of the Data Management Association’s (DAMA) Data Management Body of Knowledge (DMBOK) and Carnegie Mellon’s Data Management Maturity Model (DMM), there is virtually no structured data management knowledge available; consequently, organizations reluctantly look to professional organizations to fill the gap. Not having a qualified leadership pool is not a new phenomenon, however. One can see strong similarities with the introduction and acceptance of the chief financial officer (CFO) role. The CFO is primarily responsible for managing the financial assets of an organization. This officer is also responsible for financial planning and record-keeping, as well as financial reporting to higher management. In some sectors, the CFO is also responsible for analysis of data. Most CFOs of large organizations have finance qualifications such as a Master of Business Administration, Master of Science (MS), or come from an accounting background. They may also have certifications like Certified Public Accountant (CPA), Chartered Accountant (CA), Certified Management Accountant (CMA), Chartered Certified Accountant (CCA), or an equivalent status, such as Master of Finance. When this role was first introduced, these competencies and credentials did not exist.
Today’s CFO is singularly focused on financial matters. This person possesses the requisite knowledge, skills, and abilities to handle a wide range of financial matters from investment and acquisition to payroll and accounting. Moreover, CFOs have become an expected role within nearly every organization, and CFOs typically occupy their position for more than a decade for each job or assignment (WEBCPA Staff, 2010). The academic curriculum, training, and certification are mature and well-established by today’s standard, and organizations can be confident there is a large, qualified candidate pool when they need a CFO. Additionally, CFOs need a broad range of skills beyond the skills needed in accounting. For example, they must first be a business strategist and comfortable with technology. Built on this, CFOs develop financial strategies to increase organizational growth and profitability and create plans and opportunities to optimize the organization’s financial assets.
However, if data is truly an asset, one would expect to see similar education and credentials for EDEs, but we do not. Instead, one quickly learns that there are no formalized leadership qualifications, and there is no consensus as to what kinds of certifications are appropriate for the new EDE. Because there is no generally accepted set of educational and data management credentials, it is not surprising many organizations have trouble identifying the right person to lead the organization’s data management work. Further, because there is no accepted data management regime, finding the right person is problematic, expensive and frustrating to many organizations. Unless and until organizations realize optimizing the full value of its data requires a singularly focused, qualified, and responsible individual, organizations will not be able to change their current thinking. We need to influence the academic community to develop and offer a rigorous curriculum dedicated to training data professionals.
Data Sin No. 3: Failing to Implement a Programmatic Way to Share Data
People often ask how data fits into IT projects. This is the wrong question to ask. In a nutshell, organizations need to recognize their data requirements evolve at a rate, cadence, rhythm, and speed that is fundamentally different from IT projects. So, the question organizations must first ask is how should they incorporate data into a portfolio of IT projects? Data is different, however. Shared data must be developed, documented, and managed separately from, externally to, and ahead of all IT projects. This work is foundational, as data must be defined and stored once before it can be used, and more importantly reused, on any individual project.
The real question for organizations is where IT projects fit into data management activities. For example, IT projects exist for a short period of time to provide specific solutions to very particular business, record keeping, and analytical needs. Underlying these problems is the notion that data exists beyond any project, and data continues to evolve for as long as the organization exists. Additionally, it is important to note that data evolves at a slower, steadier pace that is different from technology. For example, an organization may have a business goal to enter a new market. The data the organization needs to achieve that goal may precede any IT project by months or even years. After the company enters the market, employees may use IT solutions to help automate certain business processes and functions. Meanwhile, the business may realize it needs other kinds of data to bolster its market position, fend off competitors, or introduce new products and services.
Even though data requirements evolve outside any particular IT project, organizations need to recognize there are appropriate times to bridge these two activities in a predictable and controlled manner. What typically happens, however, is organizations task specific IT projects with identifying the data the organization needs. When this happens, projects address their needs at the expense of the enterprise needs.
Operating this way presents an interesting problem. Projects, by definition, operate according to a finite amount of time. They have discrete beginnings, middles, and ends. Consequently, it would be wrong to assume a narrowly focused, finitely timed effort would be able to quickly, accurately, and completely identify the information the entire enterprise needs to successfully operate. Additionally, it would be wrong to presuppose these same IT projects would have the wherewithal to be able to develop the business processes and rules that would allow the organization to share its data in an unambiguous, predictable, and accountable manner.
What does make sense, though, is that this kind of work needs to be performed on a continual basis. Were this the case, data analytical work could help provide distinct input to any IT project, thereby reducing the amount of work individual projects would need to do. Working this way would also increase the likelihood of developing solutions with data specifications that are thoroughly vetted and approved at the enterprise level. The main idea is that data, its requirements, its suitability, its form, and semantics continue to evolve as the business responds to the environmental changes. In other words, organizational requirements do not stop when an IT project is complete.
It is important to note, too, that IT projects contain important motivational distinctions that sometimes work in opposition to the development of shared organizational data. Data management does not fit the IT project paradigm because data must precede any IT project. Putting it another way, the development of an organization’s data assets must occur prior to implementing a specific IT project. Additionally, organizations need to realize it will take considerable time, maybe even years, to evolve the current practices from how they work today to the way organizations need them to work. Leadership, in turn, must understand how both systems work to achieve the right balance within their organization.
A good example of this is how organizational data needs are managed. Organizations need to develop ways to better respond to changing organizational data requirements and improved understanding of existing data requirements independent of any IT project. Put simply, to leverage organizational data assets, organizations need to focus their effort on understanding their true data needs without the distractions associated with IT projects. Without this, it is impossible to accurately specify data requirements for any IT project. What needs to happen instead is that enterprise data requirements need to evolve separately from, external to, and ahead of individual IT projects. This concept is deceptively simple and direct, yet it is probably the most difficult data sin to overcome, as so many organizations are disproportionately focused on IT and hoping that IT will give them a competitive advantage.
Data Sin No. 4: Failing to Coordinate the Data Program with IT Projects
Asking data initiatives to report to IT project management creates an unsolvable and intractable conflict. IT projects are just that: projects. They have discrete beginnings, middles, and ends. However, as we have shown, organizational data must be researched, reviewed, architected, and engineered at a level above individual IT projects. IT projects are designed to do one thing and one thing alone: deliver IT solutions. Understanding what data the enterprise needs to establish or maintain a competitive position is a completely different undertaking and should be managed by an executive with a business background who is charged with managing the organization’s data assets. This is no different than what other executives do today. In other areas, executives are charged with managing other kinds of organizational assets like finances, property, and personnel, and they perform this work in an ongoing and uninterrupted manner. Those executives may spawn specific projects to accomplish things, but the overall work is a capital endeavor and continues without interruption for the life of the organization. Most importantly, IT should not be able to do anything with data without business approval.
The kind of work data executives perform is a program. Work is initiated and continues until the organization decides it no longer needs to perform this kind of work (Project Management Institute, 2001). Along the way, the organization can sponsor multiple programs, projects, and specific activities that constitute the overall data program.
The confusion surrounding data jurisdiction creates other more expensive and time-consuming problems. For example, when one looks for a root cause of poor-quality data, one quickly realizes there is a fundamental misperception about whose problem it really is. When asked, survey after survey of business professionals indicate they think data quality is performed by IT, who in turn think data quality is a function performed by the business (Aiken & Billings 2013, Aiken & Gillenson et. al. 2011, and Eckerson, 2001). Over the last 30 years, industry has conditioned people to believe data is an IT problem and that the CIO is responsible for solving it. However, CIOs must consider many more things than just data, and data quality has fallen into the crack between business and IT (Clarence & Hempfield, 2011). Research has shown that only approximately 10% of all organizations achieve a positive return on their investments in data management while about 30% achieve only substantive results (Aiken et al, 2011).
Hopefully it is becoming clear that organizations should be managing their data assets in a way that is dramatically different than they currently do. Organizations need to create a new position within the business side of the organization, which is the appropriate home for the EDE. Once understood, common organizational data needs can be maintained within the business part of the organization, and data management would be able to provide well-defined data and data requirements to individual IT projects.
In time, individual IT projects would be able to use data, metadata, and data engineering artifacts to help them at the onset of their IT work. Having data standards and specifications available to each IT project would reduce the amount of work and confusion IT projects regularly encounter. Instead, IT projects would be able to incorporate well known, well understood data plans into each IT project plan, thereby increasing the volume, scope, and utility of information given to developers. When these new processes are matured, each new IT system would be able to provide highly descriptive feedback using metadata to data management experts. For organizations to do this, however, they must first revise their understanding of data and apply that new understanding to their projects and programs. Simply directing IT workers to change the way they operate will not work; instead, this change must come from higher levels in the organization where a dedicated data management component can ensure the benefits from each specific IT project are leveraged across the enterprise. Often, this can only be accomplished by an organizational mandate.
There are, however, certain parts of data management that are shared responsibilities with IT. The Data Management Association’s (DAMA) Body of Knowledge (DMBOK) has 10 knowledge areas. The figure shows different functions and their relative alignment to the EDE and CIO. Under the DAMA model data governance, data architecture management, data quality management, document and content management, data warehousing, metadata management and data security management, business intelligence, reference and master data management, and data governance would be activities that report to the EDE. Data development and database operations management continue to report to the CIO as they are largely technology functions.
To put these ideas into context, compare data-centric thinking with traditional IT project development practices, illustrates the predicament in which data management professionals find themselves. Generally, considering data is the last activity performed after the organization creates new or identifies existing IT projects.
Using this approach, organizations produce their overall organizational strategy. The organization then defines specific IT projects that presumably help satisfy that strategy. At the end of this, individual IT projects determine what data and information that the organization uses to be competitive. Clearly, there are issues with this approach; it is actually backward. The business needs to determine what data it needs, yet the opposite typically occurs. For example, business processes and data are tightly integrated in software applications, making them difficult to maintain, change and evolve. Working under this model also means very little data can be reused with an IT-focused approach, and data requirements are focused around software applications and not the organization.
As before, the organization defines its overall organizational strategy. Next, data management must specify the data initiatives required to achieve measured goals and objectives, with an eye to organization-wide usage and derived from a shared, useful data architecture. As a result, IT projects are given definitions, specifications, and other programmatic artifacts at the onset of their work. IT is no longer responsible for performing enterprise-level analysis; instead, they can focus exclusively on providing technical solutions, which have already been aligned and normalized with an organization’s data needs. Additionally, organizations would be able to specify IT projects using the smallest possible footprint and simplest design because they would be leveraging an existing organizational data model.
This approach offers many distinct advantages over the traditional IT approach. For instance, data assets can be developed in a truly enterprise manner. IT projects would subsequently support organization-wide data needs, and their work would complement and automate various business processes. Additionally, the organization could maximize data sharing and reuse as well as reduce the brittleness associated with separating data and architecture. Taken together, this approach increases data sharing, reduces data duplication and data waste, and improves maintenance, particularly in cases where data is shared across functional areas. This approach increases data and metadata reuse and provides clearer and better understood business and technical requirements. This approach also produces more productive knowledge workers and better integration with evolving business processes and practices. In the end, it becomes clear: implementing a data-centric approach can produce fewer, less complicated, higher quality, and easier-to-maintain software and information technology systems.
Data Sin No. 5: Failing to Adequately Manage Expectations
Before an organization can take advantage of this approach, it must first do two things: (1) manage expectations and (2) align the organization to the new paradigm. It is important to note both activities are prerequisites to taking advantage of the data-centric model.
Achieving tangible behavioral changes can take years to root and mature as we have already mentioned, and it is incumbent on the EDE to ensure the organization understands how data management directly and positively affects the organization’s ability to satisfy the goals and objectives in its strategy (Aiken & Billings, 2013). Led by the EDE, the organization needs to develop corporate data management competencies in a series of chartered data management programs, projects and activities. Along the way, the EDE must set and manage organizational expectations. This means carefully balancing the changes that need to occur with delivering real-world, tangible and measurable results. Too much of one or the other will be unsustainable over the long term.
While it can be extremely challenging to show how data supports the organization’s strategy, it is equally challenging to manage expectations for new data management initiatives. If organizations want to use data as a resource, they first must understand its dualistic nature; organizations will either leverage data in support of their organizational strategy or that same data will be an impediment. There is no neutral position, and the organization needs to control the forces that would otherwise prevent it from taking full advantage of its data resources.
For organizational leaders, they need to recognize and respect that making these kinds of changes will take time, perhaps several years. The EDE needs to clearly articulate an agenda that is balanced between developing specific capabilities and measurable outcomes, and he needs to describe the agenda in a way that allows others to assign value to the overall initiative. As long as organizations maintain an IT-centric approach to their work, EDEs will have to explain why data architecture—and data management, in general—requires time to do and do well. These executives will also constantly have to defend the results of data architecture work. While organizations long to exploit big data and perform advanced analytics, organizations need to realize they must first crawl, then walk, then run relative to their data. If organizations truly want to trust the results of their computational abilities, they must be able to account for their data across the entire data lifecycle, from acquisition through final disposition.
The only way to accomplish this is for data management and information technology experts to work together as a team. As their knowledge, skills, and abilities mature, they must capture that new knowledge to guide those who follow. Consider the old adage: How does one get to Carnegie Hall? Answer: Practice. Practice. Practice. Organizations also need to realize they can’t simply go out and buy these capabilities, as tempting as this may be. Led by the EDE, these capabilities need to organic and sustainable over time. Only by understanding their existing organizational capabilities, strengths and weaknesses can they hope to achieve mastery in this area.
Data Sin No. 6: Failing to Sequence Data Strategy Implementation
William Porter’s book (1980), Understanding How to Successfully Implement Data-Based Strategies, highlighted the need to make fundamental strategic choices between innovation and improved operations. Porter suggested organizations need to understand most data management teams can’t be innovative, efficient, and effective all at once. Instead they must practice their tradecraft, realize tangible value and leverage their knowledge for future use. Not surprisingly, this explains why today’s big data projects succeed at rates comparable to IT projects (Marr, 2015) and why the recent expectations of investments in data science have produced less than stellar results (Harris and Murphy, 2013). What should be happening, instead, is for the data strategy to be sequenced and synchronized with IT projects. In practical terms, this means organizational data management practices and their maturity generally follow a progression through the four quadrants (V1 through V4).
For organizations at V1, data management is not seen as being strategically important to the organizational strategy, and organizations do little with data management that transcends individual work group levels beyond “keeping the doors open.” Moreover, the organization does not manage its data, nor does it understand data as a strategic asset. What the organization does instead is expend minimal effort toward maintaining data in order sustain its operations. The organization focuses its effort reporting cash-balances instead of developing cash-forecasting abilities.
Organizations that exist at V2 follow a data strategy that is focused primarily on increasing organizational effectiveness and efficiencies, which could be applicable for supporting lean supply chain or low-cost provider models. Organizations that exist in V3 have realized data can help them reinvent themselves and help them establish better positions within the market. A good example of this is Capital One, which is an often-cited example of such an innovator and modernizing around the idea of providing products for underserved credit populations (Lattin & Rierson, 2007). However, organizations that recognize the value of data and use it in support of their strategic plans often do so at great expense and often have less than stellar returns on their investments. Those that are in V4 are organizations that have mastered the data management practices in V2 and V3. It is important to realize most organizations require a complete reset with respect to this sin. New EDEs must use their organization’s data strategy to secure and make concrete the nature of long-term investments required to achieve their desired goals including specific capital investments in training, education, and then and only then in selected technologies.
Data Sin No. 7: Failing to Address Change Management Challenges
For organizations to leverage data as a strategic asset, organizations must mature their technical abilities using change management processes that most do not have. This kind of work is well known, however, and organizations can proceed with the necessary changes with relatively low risk levels (Keen, 1981). The key thing to remember when beginning this work is to stay focused on separating data management from IT management. If organizations do not stay focused on maintaining this separation, they will likely end up like many other quality management initiatives, failing and being labeled as just another management fad. Per management guru Peter Drucker (2006), “Culture eats strategy for breakfast.” For organizations to successfully implement the kind of changes that will help them use data as a strategic asset, they must make fundamental changes in both information technology and business. Such changes will be global and affect nearly every part of the organization, and these changes will be instrumental in securing executive sponsorship, generating momentum and realizing the business vision.
To provide a simple example of this phenomenon, consider the following: leadership is charged with eliminating the Seven Deadly Data Sins, but these people do not have the requisite knowledge, skills, and abilities to even attract, let alone hire and retain, enterprise data leadership. So even though the organization has identified a way to get past the lack of qualified data management leadership problem, the organization—and the EDE—need to address and resolve the Seven Deadly Data Sins before they try and develop smooth-running versions for their data strategy process. When organizations believe that they have complete the prelimiary activities, they need to stop, take stock of their work, and objectively measure their performance. They need to be absolutely sure that they have eliminated the Seven Deadly Data Sins before the continue to the next phase.
What happen often, however, is that individuals will begin to aggressively pursue advanced work, not realizing they have not satisfied the prerequisites. What generally happens is that organizations begin investing significant corporate capital and accomplishing little in terms of lasting change. This accounts for the vast number of EDE failures to date. In these cases, we have recommended organizations hire someone outside the organization. This person will be a “sacrificial lamb” of sorts and be the individual who endures the organizational backlash to the necessary preliminary changes. Once that work is complete, the first EDE would help hire a replacement, and the second EDE would proceed to the next set of tasks. This is what we refer to as the one-two success punch.
References
- Aiken, P., & Billings, J. (2013). Monetizing Data Management: Finding the Value in Your Organization’s Most Important Asset. Technics Publications.
- Aiken, P., Gillenson, M., Zhang, X., Raffner, D., “Data Management and Data Administration: Assessing 25 Years of Practice” Journal of Database Management 22(3):24-44 July-September 2011.
- J. Clarence and W. Hempfield. 2011. Data Quality? That’s ITs Problem Not Mine: What Business Leaders Should Know About Data Quality. Pittney Bowes Business Insights.
- H. Harris and S. Murphy. 2013. Harris 2013 Analyzing the Analyzers: An Introspective Survey of Data Scientists and Their Work. O’Reilly Media.
- Keen, P. G. (1981). Information systems and organizational change. Communications of the ACM, 24(1), 24-33.
- Lattin, J., & Rierson, M. (2007). Capital One: Leveraging Information-Based Marketing: Case Study. Standford Graduate School of Business.
- Marr 2015. Where big data projects fail. Forbes/Tech. http://www.forbes.com/sites/bernardmarr/2015/03/17/where-big-data-projects-fail/#7609662264e2.
- Porter, M. E. (2008). Competitive Advantage. Simon and Schuster.
- Guide, A. (2001). Project Management Body of Knowledge (PMBOK® GUIDE). In Project Management Institute.