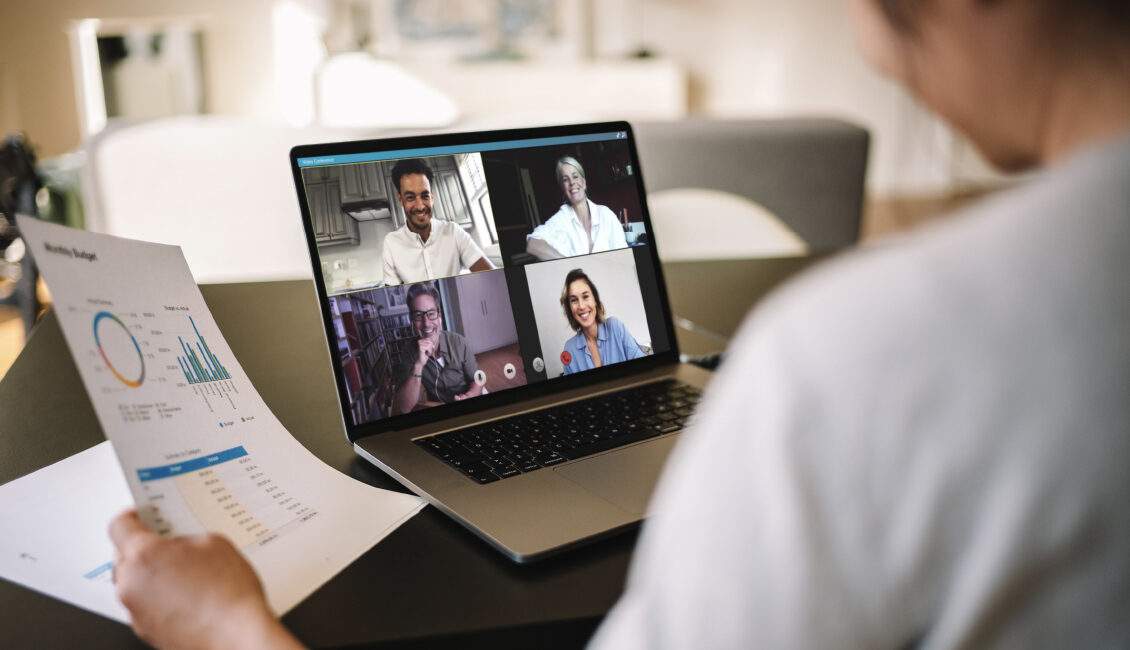
Accelerating Digital through Data Foundations
Dr. Shivaji Dasgupta, Managing Director, Head of Data and Artificial Intelligence, Deutsche Bank
Markus Mueller, Managing Director, Global Head of Chief Investment Office, International Private Bank, Deutsche Bank
Post Covid-19 economic recovery will be strongly dependent on the digital agility and ability of a company and the society in general—whether enabling work-from-home, activating online-retail or responding to the pandemic via contact-tracing, all these solutions require a strong foundation of technology and a sophisticated understanding of these technologies. Geographies that are prepared with these digital foundations, and companies that have invested early in these (or have deep-pockets currently to do so rapidly), will profit from this and can participate in a V-Shaped recovery (i.e. quicker recovery from the crisis). Countries and companies that did not and cannot invest so far, will have a difficult proposition to reverse the downward trend during the crises.
There have been two phases of digital economy in the last decade, which are disruption of the customer interface and disruption of current processes using data. In the first phase, the traditional “offline” channels were revamped via online tools—web or mobile. This has been adopted quite rapidly by almost all industries and spheres but anchoring with the offline channels is still missing in most cases. A customer calling the call-center of an airline will most likely not be able to track his request or complaints on his mobile-app for the same airline – although the check-in processes are digitized omnichannel for many airlines. Such examples are aplenty in other industries as well. Although the UI/UX challenge remains (due to lack of right skills, integration in legacy, lack of understanding of customer needs), the challenge is understood and comprehensible for most companies, and ready-to-use solutions (e.g. white label SDK for mobile-apps) resolve this challenge.
Each interaction with the customer—offline and in even more so online—leads to data-points that are valuable in offering individualized service, getting feedback and make internal processes
efficient. This has triggered the second phase of digital economy—the use of data (via advanced technologies and algorithms). Unlike the frontend, this phase has still not been completely understood (leave alone tackled) by most industries.
Not only has this magnified the transformation of core business products and services (e.g. personalization of website/mobile app, targeted notifications and beacon services, fulfilling regulatory requirements via external data-scraping), there has been a splurge of new platform business models. These are businesses utilizing network effects between (white label) service producers and consumers. Marketplaces like Amazon, Search platforms like Google Search, Video-tainment platforms like Netflix are examples for such businesses, and the underlying differentiator for these businesses is the way they are using their data. Netflix for instance produces shows based on user preference of story, cast and attention span—and so the very popular series “House of Cards” was created.
Capitalizing on Data
Two of the main technology foundations that are at the core of accelerating digital ways of working with data are artificial intelligence and cloud technology.
ARTIFICIAL INTELLIGENCE (AI) is the science of recognizing patterns and offering intelligent recommendations by a machine-algorithm based on (historical) data collected. The larger the volume, the wider the variety and the quicker the velocity of the data being fed into the algorithm, the higher the accuracy of these recommendations. Two ways to implement AI are either to build the algorithms based on tailored data, or to implement off-the-shelf generic algorithms and invest in training with in-house data (also known as low-code/no-code solutions, e.g. Dataiku, Alteryx). Use of either solution depends on the task at hand, i.e. the use-case for AI. Readymade solutions for automatic chatbots, individualized website design via personalizer and even stock-trading solutions are available aplenty in the market and need not be built again; on the other hand, an end-to-end claims processing algorithm for insurance would require specific customization based on depth of services being offered, etc. Both these approaches require the data to deliver the required results.
CLOUD TECHNOLOGY allows access of modular and on-demand software and hardware resources without large bureaucracy via internet. This technology is pivotal for the platform business models to offer “everything-as-a-service”, because they require the flexibility and scaling of costs. A big argument around cloud is conversion of the CapEx costs to OpEx costs via pay-as-you-go models.
Cloud encompasses three big features for any tech-operations—
- Infrastructure as a Service (IaaS) allows data hosting solutions virtually. IBM, Oracle, DXC have been long existing players in this field, having helped on-premises technology to be virtualized
- Software as a Service (SaaS) allows cloud-based software to be used for running in-house operations. All users profit from the improvement in software via crowd-sourcing. IBM Watson Suite, Microsoft 360 are applications in this category.
- Platform as a Service (PaaS) allows data hosting and use of cloud based software for the holistic use and adoption of cloud technology. 3 big hyper-scalers currently dominate the market – Amazon Web Services, Google Cloud Provider and Microsoft Azure.
Interestingly, the three hyper-scalers in the US (and Alibaba Cloud from China) have a few things in common. They all started their businesses nowhere close to be a cloud-provider but rather providing platform services to end-consumers (Microsoft being the exception, they produced their own services but has transformed their own business model marvelously in the past two decades). Providing platform services, these companies are very quick in gathering tremendous amounts of data—which they effectively started using (and pioneering) with the most advanced analytics and AI algorithms. Search-engine-optimization and predictive next-best-offers are some examples of these. They realized that they can capitalize on their experience with storage/handling of large sets of data by offering these as cloud services and their revenue share with cloud has skyrocketed. Once they have established their lead using AI tools with best-in-class analytics, they are offering these either as pre-included SAAS tools or managed services on-top.
Recent advances in the field of quantum computation has allowed the testing to be broadened in commercial use-cases. This is a technology that exploits different quantum energy levels for simultaneous computation, increasing the ability to process and tackle min. 2-fold larger sets of data and increasing the speed of processing the algorithm by at least 4-fold. This enables solving complex optimization problems (e.g. FX arbitrage), rapid system simulation (e.g. risk analysis) and improves cryptographic capabilities due to quantum entanglement techniques that allow data encryption. This technology is currently very nascent and commercial roll-outs are not expected in the next 3-5 years. There are features though that are very interesting and usable for large-scale implementation, especially in relation to encryption. This can be used for secure data transfer (cyber security) as well as compliance to privacy regulations for analytics (homomorphic encryption).
The investment for research on these topics is quite enormous, and it is unlikely to realize on-premises implementation of quantum computers. Rather cloud-based integration of quantum computers is a much more likely scenario, for corporations/states using the cloud facilities to access quantum features. And here too, the chances are very high that the hyperscalers will be leading the integration of this technology into their cloud services.
Adopting AI Via Cloud
AI is the game changer for digital business-models. Similar to scale effects for mass production and globalized outsourcing for cost reduction, AI will be key for hyper-personalization to fulfill individual needs while being efficient. Beyond personalization, AI will also enable developing ecosystems by bringing together data from networks of customers, clients and 3rd parties. Also, the use of AI in defense and cyber-space will determine the cutting edge of geographies over each other, due to machine-driven precision activities compared to human heavy, high-cost planning. Compared to linear evolution of services via automation, AI adoption leads to a revolution of the business model with mostly non-linear improvements due to multifold effects on higher reach (i.e. revenue) and higher efficiency (i.e. lower cost) at the same time.
Adopting PaaS technologies accelerates the AI journey manifold as
- cloud hosting technology allows large volume, wide variety and fast velocity of data to be scaled easily due to virtualization
- virtualization also allows modularized use and hence, cost elasticity
- cloud-based software tools are open-source based, and hence, easily adaptable and manageable
- improvements to cloud-based software is rapid due to crowdsourcing (e.g. Google’s Tensorflow), and many ready-to-use algorithms readily available
- many low-code/no-code tools available increasing the usability and reach of AI
- pre-integration to data on the platform allows for easy consumption
Cloud-based AI adoption is not a linear improvement compared to on premises implementation but has a rather huge exponential impact. Data storage on the cloud scales in cost in a non-linear, negative manner, meaning the larger the volume of data being stored on cloud, the lower per byte are the costs for storage. Similarly, licensing of software in a PaaS environment is extremely elastic and costs do not explode with multiple simultaneous usage. This coupled with the benefits mentioned above, leads to an exponential increase in revenue.
Adding quantum computation to the equation, there is an even stronger acceleration of AI roll-out, and thereby digital business models expected. Due to the encryption features, implementation of analytics and AI can be expanded much easier to all aspects of data; also, the possible use of quantized levels enable data processing for enormous volumes in very short time.
Reality already but slow integration
The intersection of these technologies is reinforcing extreme transformation of the data stack for multiple companies – from heavy use of internal technology stack for data (for collection, connectivity via API, analytics, etc.), there is a shift towards PaaS-based virtualization. Especially for real-time data and exploitation of new technologies e.g. 5G-enabled IoT devices will be a necessity. This transformation is already leading to a revolution of customer interaction from current digital tools like mobile and web-based interfaces to AR/VR, Voice-based interfaces.
There are some financial service providers such as Temenos that is partnering with Google Cloud and Mastercard is partnering with all 3 hyper-scalers making this transition tangible. In the energy sector, Engie has dived into cloud integration and scaling AI based on its vast assets of data from gas turbines, launching self-service applications for customers as well as employees and also, engaging in conceptualizing smart-cities.
Additionally, the technological advances are allowing financial regulation to be implemented more transparently. Especially, with quantum computational techniques of entanglement and encryption 7 the privacy-enhancing techniques may become a mandatory part of data sharing. Therefore, it is imperative for companies and states to adapt their digital capabilities and adopt these technologies to digitize and stay relevant with their current business/delivery models. A new technology stack for fully utilizing data is necessary – encompassing cloud technologies and AI. In case of shifting to platform business models, this transformation becomes even more pressing to leverage the network effects.
Reference:
- Platform Economy (Geoff Parker, et al.)
- Digital Transformation (Thomas M. Siebel)
- World Economic Forum AI Discussions